We are pleased to announce the selected performers.
Quantum computing promises to have wide-ranging impact on many aspects of our lives including our health. But how? And when? These are the questions often asked and the ones that generate both optimism and skepticism about quantum computing applications. We do know that the early days of any new computational method benefit from the co-development of application, software, and hardware – allowing early optimizations with not-yet-generalizable, early systems. And history has shown us that the disciplines that begin this interaction early are also ones that advance and benefit most. The Wellcome Leap Quantum for Bio program aims to accelerate applications of quantum computing in health and demonstrate quantum-enabled solutions to address pressing human health challenges.
The power of quantum computing stems from the unique properties of quantum systems, notably: superposition, interference, and quantum correlations. When these properties are exploited for information processing, quantum computing enables solutions to problems that are not practical using classical computers. Examples of such problems include finding the prime factors of large numbersi (the basis of most public key cryptography methods), high-accuracy quantum chemical calculations (the cornerstone of drug designii, iii), and unstructured data base searchiv. Quantum algorithms for these problems and other industry-specific applications (e.g., elucidating the reaction mechanism of nitrogen fixation in nitrogenasev or calculating the electronic structure properties of hemoproteins to elucidate drug-drug interactionsvi) offer exponential or polynomial speed-up compared to their classical counterparts and require quantum computers at scales not realized to date.
Inspired by the promise of quantum computing, strides are being made toward large-scale quantum computers capable of outperforming even the fastest supercomputers possible. Notably, in 2019, a quantum computer based on superconducting circuits performed a mathematical benchmark task on a 253-dimensional computation state-space in 200 seconds that would have taken over 10,000 years on the most powerful classical computers available at the timevii – experimentally demonstrating for the first time the exponential speed-up between a quantum and a classical algorithm. But because quantum computers are inherently sensitive to outside perturbations, scaling them up is a considerable technological challenge. Today’s quantum computers remain limited in size and computational power, which in turn limits their practical application. For example, the largest quantum chemical calculation with chemical accuracy performed on a quantum computer to date is finding the binding energy of a 12-atom hydrogen chain (H12)viii, a problem within reach of classical computers.
One promising area where quantum computing could deliver near-term practical advantage is in human health applicationsix, where biological data can be represented more efficiently on a quantum computer compared to a classical computer. For example, the haploid human genome is around 3.2 billion base pairs long and can be represented by 6.4 x 109 classical bits or around 750 MBx. In quantum computing, the analogue to the classical bit is a quantum bit (qubit), that can be in a superposition state between 0 and 1. A quantum register with N qubits is in a superposition of 2n possible states with amplitudes, where each can encode a classical bit. Hence, it is in principle possible to store the entire human genome in just 33 qubits, while less than 100 qubits would be required to store the genetic information of every living person on the planet.
Indeed, sequencing of the human genome led to the generation of genomic data that is predicted to reach between 2 and 40 exabytes within the next decadexi, and 3D protein structure prediction involve similarly large and expanding data sets. These technological developments require the application of statistical and machine learning methods for data mining to make predictions relevant to research and clinical work. In drug discovery, computationally intensive methods are used to forecast molecular properties, ligand-protein binding, secondary structures, and more. For such applications, quantum machine learning algorithmsxii promise to outperform their classical counterparts and enable handling larger data sets.
More than 40 experiments on proof-of-concept quantum computing applications in human health have been carried out in the last few years, including applications in clinical research and discovery, diagnostics, and treatments/interventions such as ligand-protein interaction energy calculations via a hybrid quantum-classical approachxiii, medical image classification via quantum neural networksxiv, and studying the mechanism of biological catalysts that play an important role in breaking and forming of chemical bondsxv. To date, however, none of these methods have produced substantial quantum computing speed-up compared to high-performance classical computing for industry-scale problems.
Two parameters define the capacity for problem solving of quantum devices, jointly referred to as quantum computing resources: first, the number of qubits, which determine the size of the computational space; and second, computational runtime, otherwise known as program depth. In Fig. 1 the quantum computing resources required for several applications are shown in log-log scale together with the capabilities of current quantum computers. There is a gap between quantum hardware and algorithmic resources that needs to be closed – doing so will illustrate the beginning of useful quantum computing and there is now a race toward demonstrations of practical quantum advantage for near-term quantum devices.
Wellcome Leap’s Supported Challenge Program in Quantum for Bio is focused on identifying, developing, and demonstrating biology and healthcare applications that will benefit from the quantum computers expected to emerge in the next 3-5 years. Up to $40M in research funding will be awarded to multidisciplinary, multiorganizational teams and up to $10M in challenge prizes will be available at the end of the program for successful proof-of-concept demonstrations on quantum devices with a clear path to scaling to large quantum computers.
Notably, we are interested in the development of algorithms that will be realizable on quantum computers expected to emerge in the next 3-5 years; estimated to be able to execute programs with 100 to 200 qubits and depths of O(105-107), which we refer to as the target resources.
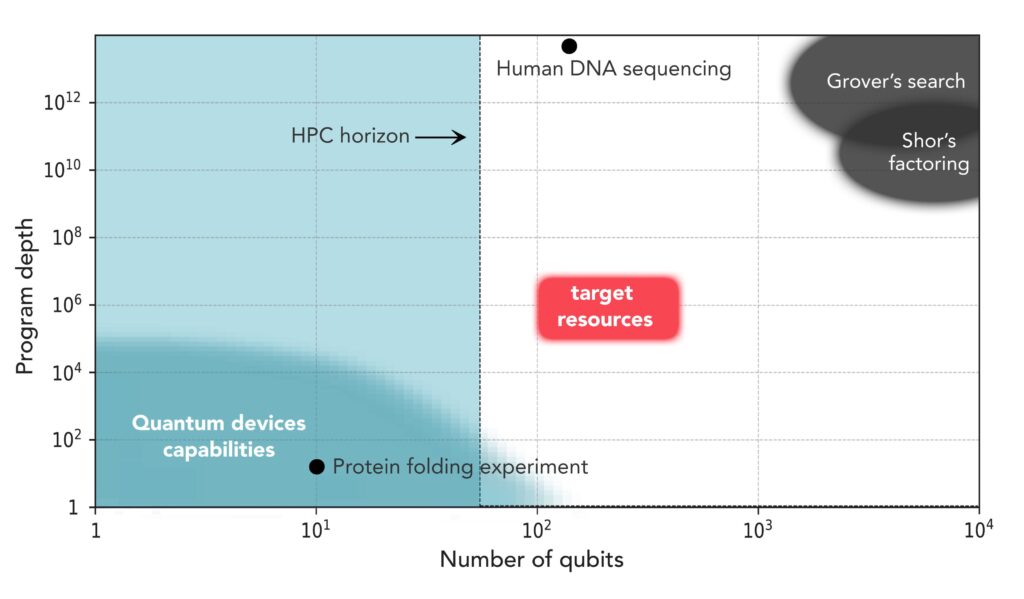
Examples of areas of interest.
We are soliciting proposals for algorithmic solutions to human health problems whose quantum computational resources fit into the target resources box in Fig. 1. As an example, quantum search algorithms can be used to perform pattern matching for DNA sequence alignment with a polynomial algorithmic speed up compared to classical, direct brute-force search algorithms. The quantum Grover search algorithm for human genome assembly with short reads of 50 base pairs lengths is estimated to require 133 qubitsxvi. However, the estimated program depth for this task is O(1014-1016), which translates to days and weeks of runtime. This is beyond the capabilities of the quantum devices that will be available in the next 3-5 years. To enable quantum speed-up for sequencing on near term quantum computers, we need to develop more efficient information encoding, decoding, and processing methods, thereby reducing the number of required qubits, which in turn will reduce the depth of the algorithm.
Another representative example is physics-based ab-initio prediction of the three-dimensional structure of a protein from its primary sequence of amino acids. Sampling the conformational space of proteins is intrinsically NP-hard and classical ab-initio protein folding algorithms have been replaced by deep learning algorithms with good but not perfect accuracy and applicability. The benefit of using quantum computers stem from their ability to efficiently sample the exponentially growing conformational space, which will extend the applicability and accuracy of protein folding predictions and shed light on the mechanistic process itself. Early attempts at formulating the protein folding problem for small, noisy quantum computers showed a model Hamiltonian with O(N4) scaling for a polymer chain with N monomers on a lattice, while the number of qubits scales quadratically with N. This hybrid algorithm was implemented experimentally on a quantum computing device with 9 superconducting qubits and program depth ~O(101) for the study of the folding of a 7 amino acid neuropeptidexvii. The most abundant proteins in the human body have lengths of several hundred to dozens of thousands amino acids. In order to benefit from the power of quantum computers for larger proteins however, we will need novel approaches for qubit and program depth minimization. Additionally, we will need more realistic descriptions of the amino acid side chains and a more systematic treatment of the long-range interactions.
Format of Wellcome Leap’s Quantum for Bio Supported Challenge Program.
The program will take place over three phases as diagrammed below:
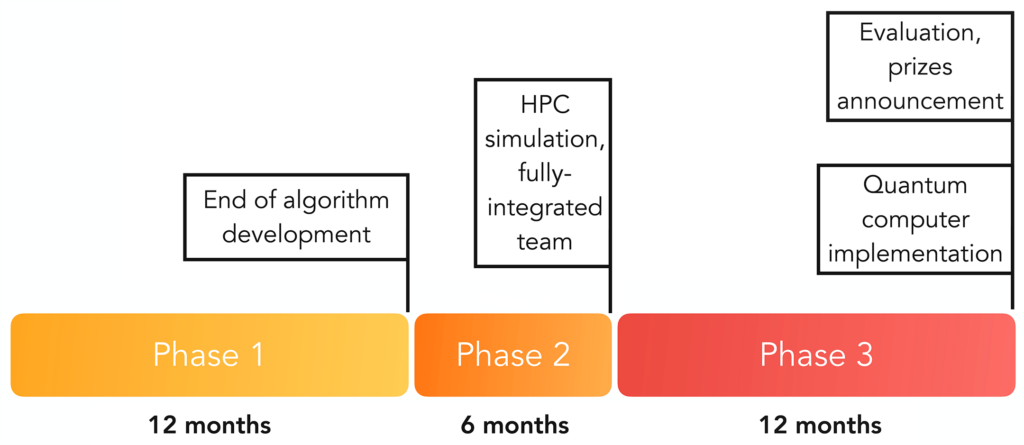
Phase 1: A maximum of 12 cross-disciplinary proposals will be selected for funding and will participate in Phase 1. Each team should include expertise in human health and quantum algorithm development – quantum hardware expertise is encouraged but not required for this phase of the program. A maximum of $1.5M in funding will be awarded to each team selected for funding in Phase 1, which will be twelve (12) months in duration.
The focus of Phase 1 is quantum algorithm development where the quantum computing resources (number of qubits and program depth) required should fit within the target resources box in Fig. 1. The technical progress and deliverables of Phase 1 will be evaluated and tracked by the Wellcome Leap Quantum for Bio Program Director (PD) and an expert internal technical team. The final evaluation of Phase 1 will be conducted with the help of 4 additional, external subject matter experts in quantum computing and human health. Teams that are deemed to have demonstrated a significant advance for human health within the defined target resources will proceed to Phase 2.
Phase 2: The focus of Phase 2 is large-scale simulations of the developed algorithms in Phase 1 using classical high-performance computing (HPC). Teams will be awarded a maximum of $500K in funding for HPC expenses and up to $250K for other related technical and programmatic execution expenses. Phase 2 will be six (6) months in duration and at the end of Phase 2, all teams will be required to have identified and secured quantum hardware expertise participation on their team in order to be considered for progression to Phase 3.
Progress in Phase 2 will be evaluated and tracked by the Wellcome Leap Quantum for Bio PD and technical team. To successfully complete Phase 2, teams will perform a classical HPC simulation of their quantum algorithm for 30 to 40 qubits and compare the results to the ones obtained by the standard classical approach for the respective application. Wellcome Leap’s internal technical team aided by external HPC subject matter expert(s), as needed, will decide which teams successfully complete Phase 2 and proceed to the final Phase 3.
Phase 3: The focus of this phase is implementing the developed algorithmic solutions on quantum computing devices. A maximum of $2M in funding will be awarded to the fully integrated teams for Phase 3, which will be twelve (12) months in duration. Phase 3 technical deliverables will be evaluated and tracked by the Wellcome Leap Quantum for Bio internal team as a condition for entry to judging for prize awards. The final evaluation of the Wellcome Leap’s Supported Challenge Program results will be conducted by an integrated team that consists of the internal technical team and 4 or more external subject matter experts in healthcare and quantum computing.
Prizes.
A $2M prize will be awarded for each team that successfully demonstrates an experimental realization of their application on a quantum computer with more than 50 qubits and a program depth of O(103-104) and a clear path to scaling to larger quantum computers.
A $5M grand prize will be awarded to one team that successfully executes their algorithm on a quantum computer using quantum resources that fit into the target resources box in Fig. 1. In case there is more than one team that achieves this goal, the team of experts that perform the final evaluation will award the grand prize to the team whose application is deemed to be most significant for advancing human health.
Program Director.
Elica Kyoseva, PhD has expertise in quantum computing and its applications to drug discovery.
She was a Fellow at Massachusetts Institute of Technology, a Marie Curie Fellow at Tel Aviv University, and served as Entrepreneur in Residence and Advisor at a venture capital firm. Most recently, she worked at Boehringer Ingelheim as a quantum computing scientist. She earned her PhD in Quantum Optics from Sofia University, Bulgaria.
Who are eligible Wellcome Leap program performers?
Eligible performers are from universities and research institutions: small, medium and large companies (including venture-backed); as well as government or non-profit research organizations. We encourage individuals, research labs, companies, or small teams to apply in program areas best aligned with their expertise and capabilities.